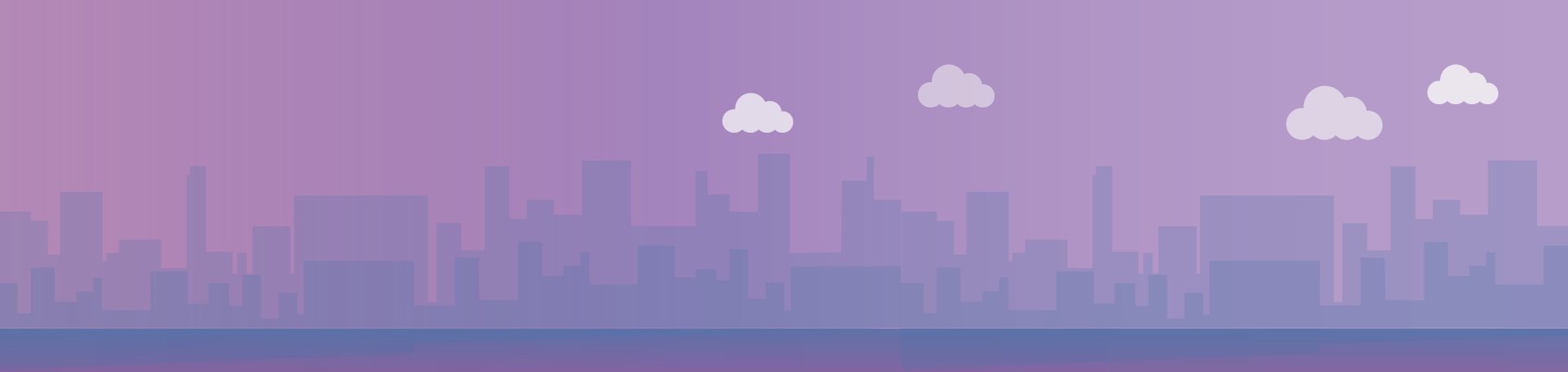
Implementation Best Practices for an Efficient Transaction Monitoring System
There are four key phases in transaction monitoring (TM) implementations and how a bank should design and execute these phases. Successful implementation of rule selection, data prep, segmentation, tuning and operational optimization will determine the success of the overall TM implementation in your organization.
You can also view our on-demand webinar here.
1. Selecting and Implementing ‘Smart’ Detection Rules
Whether you are upgrading your TM to a new version or implementing a brand-new system, this is a good opportunity for your bank to revisit the current rules selection, identify any existing coverage gaps and prepare a plan to mitigate those gaps in the new platform.
Instead of following an “Out Of Box” (OOB) or like-to-like implementation, you should carefully evaluate which AML risks are applicable for the bank and what type of models and rules should be defined in the new platform to be able to provide comprehensive coverage for suspicious activity monitoring. Selection and implementation of smart detection rules are at the heart of a successful TM implementation project.
A project can have hundreds of OOB rules, but how to determine which rules to implement and which ones not to implement is key to assuring risk mitigation, timelines, efforts and costs are kept at bay. The decision should be based on your risk and coverage assessment findings.
The bank should start with:
(1) Identifying the customers who are most likely involved in money-laundering
(2) Evaluating how those customers carry out transactions and which typology they use for fund transfers
(3) Finding out where customers are sending their transactions to and from as far as countries and geographies
(4) Examine which products they are using for carrying out money laundering and terror finance transactions
When combining these elements together, the bank will be able to create a typology matrix that will serve as the foundation for mapping the bank’s risks and turning them into rules. The typology matrix will also be helpful in determining whether OOB rules will fully mitigate the identified risks or whether additional targeted rules should be developed.
Once you have the list of scenarios, we highly recommend prioritizing the implementation of the rules based on severity, data availability and business objectives. Rather than performing the rule deployment all at once, we suggest to do it in batches. A phased methodology will help the implementation team face challenges along the way and to prepare a mitigation plan for following phases of deployment.
2. Data preparation and Execution
Data drives the success of the implementation process. If the bank does not currently have a comprehensive technology layer performing a comprehensive daily routine to assure quality and exception case capture the rest of the exercise of implementation or upgrade will prove to be of partial success and results.
In the past, banks used to work in silos, where each department had its own version of data in its staging area. Within the modern programs of most financial institutions that is no longer the new norm. We encourage customers to consolidate the data required (structured and unstructured) for different compliance functions into one centralized financial crime enterprise data hub. The hub will be a master source, serving different groups such as AML, fraud prevention, reporting, analytics and modeling within the organization. Over time, some organizations may even find this golden source to have an additional use for other departments within the firm.
Instead of creating siloed data environment for different applications, we encourage customers to look at what OOB data hub functionality is being offered by the financial crime prevention software provider. For example, NICE Actimize offers a unified data model that was built based on “source once and use for all applications” principle, where customers can source all data required for all applications into a unified data model. The picture shows how Actimize detection and case management applications are closely integrated to work with a unified data model.
As an example, Matrix-IFS is currently helping a customer who is working on a multi-year AML transformation roadmap, building a centralized data environment in the cloud. The goal is to bring all sorts of data into the cloud’s Data Lake so that the bank will be able to deploy additional advanced analytics techniques (such as unstructured data) or to develop a dashboard to visualize how customers behave across different touch points with the bank, across regions and in use of certain products or services.
Data Quality Automation
A key aspect to the data related to the program is the quality which can be obtained. In addition to building a comprehensive holistic data environment, the bank should also build adequate data quality, data lineage, and data model metadata controls.
Assuring data quality validations are conducted manually or event scripted for pre-production (“Day One”) is typical and easy to achieve. However, assuring the continued quality of data day-to-day post-go-live is far more complex and requires discipline. If you are not using an automated data monitoring system you will struggle to achieve this goal. Many firms may already have simple routines to capture whether a daily data file arrived or not, and consider that the only validation required. Our recommendation for customers is to have a much more comprehensive and automated data quality program. The program should include granular validation on a table and field level, having mechanisms to turn around fixes and have remediations for those exceptions when they happen within minutes and hours (“live”) – not a month after the fact. Thus, ensuring the quality of data is an on-going responsibility.
3. Intelligent Segmentation & Tuning
Segmentation and tuning are two areas that have been going through a significant transformation in recent times. With the advancement of Machine Learning techniques, banks are able to create more granular intelligent segments of customers by leveraging transactional data, KYC attributes, client risk profiles, product usage, and more.
When upgrading the existing solution, banks should move away from the traditional approach of creating segments using static KYC attributes such as customer type and customer category. Combining smart detection rules with activity based intelligent segmentation and risk-focused thresholds will result in high-quality alerts and reduced false positives.
One additional good “Tip” for banks, is to build a sandbox environment for carrying out periodic tuning activities. The process in the diagram below shows the typical end-to-end steps involved in the tuning process.
4. Operational Optimization
As we all know, the consumers of the transactions monitoring system outputs are the AML Operations teams and the end-user investigators. By configuring the solution’s intelligent segmentation and tuning tools, the implementation team should be able to cut the false positive alerts (“Noise”) being generated out of the system.
However, even after the tuning process is complete, you are still likely to have false positive alerts. Instead of spending too much time on manual investigative processes for false positive alerts, we recommend that the bank looks into leveraging new techniques, such as consolidating alerts, workflow optimization and Robotic Process Automation to reduce manual processing times associated with (but not limited to) data gathering, excel processing, third-party system access. The integration of FIU and AML investigations workflow optimization with intelligent automation, will not only help to make investigations consistent but will also help to reduce the operational cost for the bank.
For more questions on implementation best practices, regardless of the platform please contact us using the form below. That includes migrations, upgrades or new implementation of any financial crime or data solutions.
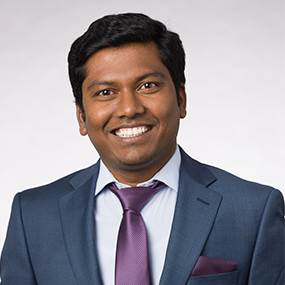
Written by Varatharaj (Raj) Sankararaj - Managing Director, Financial Crime
Find out more
Please complete your details and we will contact you
Enter your contact details
Please submit your details
Enter your contact details
Please submit your details